Economisch weerbericht (114): Big Data als nieuwe levensader
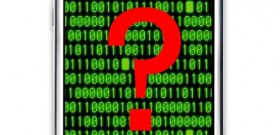
Data vond T.S. Eliot vast niet poëtisch genoeg. Wel leed hij overduidelijk aan het leven, toen hij na de Roaring Twenties, midden in de Great Depression en aan de vooravond van de Tweede Wereldoorlog zijn dichtader als volgt liet vloeien:
Where is the Life we have lost in living?
Where is the wisdom we have lost in knowledge?
Where is the knowledge we have lost in information?
(The Rock, 1934)
Met de onstuitbare komst van Big Data —geen giga- of terabytes, maar peta-, exa-, zetta- en uiteindelijk yottabytes—hebben Eliots regels ruim driekwart eeuw later alleen maar aan actualiteit gewonnen. Toch vraag ik me af: data > informatie > kennis—wat is er meer dan deze trits? Wijsheid? Ik waag het te betwijfelen.
Wijsheid
Wat is wijsheid? Dagelijks horen we mensen zich dat afvragen—meestal voor de vorm. Ik zal het u zeggen: wijsheid is vooral inzien, dat hopen extra data altijd leidt tot meer informatie en meer kennis, maar dat hun succesvolle toepassing daarmee lang niet altijd is gegarandeerd. Hoe zit dat?
Data verouderen makkelijk, ze kunnen simpelweg onjuist zijn of niet de juiste. De klok tikt om te beginnen altijd door; alles om ons heen verandert continu. “Panta rhei”, de oude Grieken wisten het ook al. Het is geen diep filosofisch inzicht, maar een fundamenteel gegeven: basiskennis zogezegd. Filosofie was toen kennisvergaring, wetenschap avant la lettre, en heel praktisch bedoeld. Laten we er nog een paar clichés en tegeltjeswijsheden bovenop stapelen en kijken waar we uitkomen.
Kopzorgen
Sinds mensenheugenis weten we dat data ons letterlijk hoofdbrekens opleveren. En kopzorgen als we afgaan op gegevens die om de een of andere reden niet kloppen. Geavanceerde hulpmiddelen als relationele databases, data warehousing en data mining op snelle computernetwerken moeten zorgen, dat we op basis van de juiste data op het juiste tijdstip de juiste beslissingen nemen. Maar jaar in jaar laat het topmanagement van organisaties desgevraagd weten niet te vertrouwen op de informatie waarover ze beschikt. Kennis is macht, maar er is slechts informatie. Onbetrouwbaarheid even daargelaten, geeft die informatie misschien kennis van zaken, maar dan moet wel eerst alles bij elkaar worden opgeteld, om het simpel te zeggen.
Risk society
Bij repeterende omstandigheden volstaat misschien ervaring plus niet te vergeten een gezonde dosis intuïtie, omdat, zoals dat heet, de geschiedenis zich wel herhaalt, maar nooit op dezelfde manier. Bovendien, en dat is een basiskenmerk van onze Risk Society, lijken eerder behaalde resultaten tegenwoordig steeds minder garantie te geven voor de toekomst.
Als we dit alles op onze vingers kunnen natellen door een aantal algemeenheden achter elkaar te zetten, hoe moet het dan wel niet zijn met Big Data—met peta-, exa-, zetta- en yottabytes aan “whatever” data? Het antwoord laat zich raden: het is bar en boos. Vanuit de eScience weten we vandaag dat Big Data vooral uitdagingen met zich meebrengt. De volgende opsomming van uitdagingen—“complexity, change, volume, demand, quality, energy & theory”—met betrekking tot “Data-Intensive Research” is van mei 2010:
= = =
Current research challenges
Complexity
The data that is used is complex for many reasons:
(a) the phenomena studied are themselves complex having many different properties that are observed separately;
(b) the analysis is complex in terms of the set of steps required and the computational complexity of some of these steps;
(c) the means of collecting data and of modelling phenomena are many and varied; and
(d) data originates from different autonomous groups who collect data without knowledge of its eventual use.
Our database theory and technology has been made tractable by imposing a discipline on the structures used and on the forms of data and processing that are considered by the machinery. It is evident that the new requirements take database theory and technology out of this comfort zone.
Change
There are several forms of change that need to be accommodated:
(a) the continuous or periodic acquisition of new data takes a different form from transactional systems—it is predominantly an addition process of primary data with complex consequential changes to derived data;
(b) the required structures evolve as the understanding and scope of the data-intensive research evolves; and
(c) new users, new uses and new alliances change the set of data that must be accommodated and the patterns of use.
As the technology supporting the databases is extended to meet complexity requirements, scaled-out to meet volume and demand requirements, and is interconnected in distributed federations, it becomes progressively more diffcult to deliver an agile system that can accommodate change quickly enough.
Volume
(a) There is a straightforward challenge of storing sufficient data economically.
(b) There are significant challenges in moving large volumes of data and
(c) a pressing need for effective incremental systems for maintaing replicas and derivatives.
(d) Whenever the analysis algorithms require access to large proportions of the data then it is necessary to use balanced architectures that give best throughput for given expenditure and energy.
(e) Many of today’s analysis algorithms cannot be applied to large data volumes and a family of incremental alternatives are needed.
Demand
The increase in demand takes two forms:
(a) more users are making requests against the collections of data, and
(b) as users become familiar with the power of data-intensive methods they ask more and more sophisticated questions.
These combine with the challenges outlined above to demand
(a) better engineering to support the required throughput and response times economically, and
(b) better mechanisms for forming and submitting questions that give good quality feedback to enable iterative improvement of requests, so that there is less increase in the demand for expert help.
Quality
Much of the legacy data and many of the incoming streams of data suffer from data errors and omissions. Many of the data collection processes sample in such a way that they are prone to sampling artefacts. In some cases, they are historic data where there is no opportunity to improve the quality. These raises challenges such as:
(a) how to build technology that is robust in the presence of such errors;
(b) how to discover and communicate issues regarding the quality of the data that may have affected a user’s request; and
(c) how to compose quality information to indicate the reliability of derived results.
Energy
The present strategies for data storage and data-intensive computation and communication will hit an energy wall if the increasing volumes and demands are met by scaling out the existing technology.
Theory
Database theory describes a critical part of the domain under discussion but it does not combine well with the theory underpinning most data-analysis methods, has not considered all of the complexities introduced above and may not yet have the capacity to structure the discussion of the change, volume, demand and quality challenges enumerated above. The theme may be able to sufficiently clarify some of these challenges so that database theoreticians can engage.
The theme can only contribute from the database point of view to aspects of these pressing challenges. There are many other challenges, such as the social and ethical issues, preservation and durability, provenance, etc. that the theme will not consider.
= = =
Agnostisch
Voor de goede orde: “the theme” is hier sysnoniem met “Data-Intensive Research Theme”, zoals het proposal heet. Wat we hier lezen, zijn feiten, harde kennis, die behalve voor de wetenschap—want data is “agnostisch”, zoals dat zo mooi heet—ook geldt in businessomgevingen en alle databases die daar een rol spelen tot aan de “prediction market value” van digitale sociale netwerken en het “Web of the World” toe. Onder “Web of the World” verstaan we het digitaal-fysieke “multibillion node, real-time sociotechnical network of humans and sensor-rich mobile devices” waarin “people, places, times, objects, activities, interests & events” samenkomen. Het zijn de digitaal-fysieke lifecycles, de real-life value chains in de wereld van morgen, laten we zeggen van 2020.
Het nieuwe Smart
In dit ultieme “ecosysteem” zou je misschien zeggen, dat we in principe alles zouden moeten kunnen weten, maar wie een beetje nadenkt, voelt op zijn klompen aan, dat dit juist grote onzin is. Immers waar moeten we beginnen, hoe snel verouderen de gegevens en hoeveel potentiële gouden bergen aan informatie moeten we niet laten liggen, vanwege de onhandelbare hoeveelheid van recombinaties?! Er is geen beginnen aan, maar we hebben het over ons afgeroepen en weten tegelijk: “The Answers Are Hidden in the Data”, “Welcome to the Decade of Smart”. Doorstoten naar het nieuwe “Smart” is zonder dat we precies weten waar we het over hebben de opgave—of het nou gaat om financiële markten, wetenschappelijke doorbraken, klimaatoplossingen of “doing business in a smarter way”.
Crap en ruis
Big Data verwerken en uitnutten is vooral een westie van “cut the crap”, van signalen oppikken temidden van alle ruis. Dat moeten we leren, zoals we de hele geschiedenis door hebben moeten doen. Betere algoritmes, betere patroonherkenning, noem maar op. En wel graag real-time, want achter de feiten aanhollen zijn we met alle crises in onze Risk Society van de afgelopen tijd wel een beetje heel erg beu. Dus als T.S. Eliot—want zo kwam ik erop—de geboortige Amerikaan, die zich veel meer senang voelde in het Engeland van oude waarden en tradities, tegen alle hektiek van de jaren ’30 in deze drie wereldberoemde regels op papier slingert:
Where is the Life we have lost in living?
Where is the wisdom we have lost in knowledge?
Where is the knowledge we have lost in information?
—dan lijkt het alsof we een “heelheid” van hogere authentieke waarden zijn kwijtgeraakt in de profane actualiteit van onze sociaal-economisch-technologische vooruitgang. Dat mag zo zijn maar het antwoord is met een lange Eliotiaanse zin, dat we ons in deze Decade of Smart—die nog gerust langer zal duren om tot overtuigende wasdom te komen—dat we in die sociaal-economisch-technologische transitiefase via idealiter een nieuwe “Age of Discovery”, annex een nieuw Humanisme en nieuwe Verlichting een heel end op zullen schieten in de richting van misschien niet een Singulariteit, maar op basis van Big Data toch in elk geval een “Web of the World”, het digitaal-fysieke “multibillion node, real-time sociotechnical network of humans and sensor-rich mobile devices” waarin “people, places, times, objects, activities, interests & events” hopelijk zinvol beginnen samen te komen als “the Life we have lost in living”. Als een streep onder al het noodzakelijke gemier van de afgelopen twee eeuwen sinds de start van het Victoriaanse internet.